Much research aims to detect online radical content mainly using
radicalisation glossaries, i.e., by looking for terms and expressions associated with
religion, war, offensive language, etc. However, such crude methods are highly
inaccurate towards content that uses radicalisation terminology to simply report on
current events, to share harmless religious rhetoric, or even to counter extremism.
Language is complex and the context in which particular terms are used should not
be disregarded. In this paper, we propose an approach for building a representation
of the semantic context of the terms that are linked to radicalised rhetoric. We
use this approach to analyse over 114K tweets that contain radicalisation-terms
(around 17K posted by pro-ISIS users, and 97k posted by “general” Twitter users).
We report on how the contextual information differs for the same radicalisationterms
in the two datasets, which indicate that contextual semantics can help to
better discriminate radical content from content that only uses radical terminology.
The classifiers we built to test this hypothesis outperform those that disregard
contextual information.
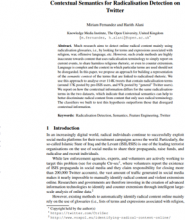
Contextual Semantics for Radicalisation Detection on Twitter
x