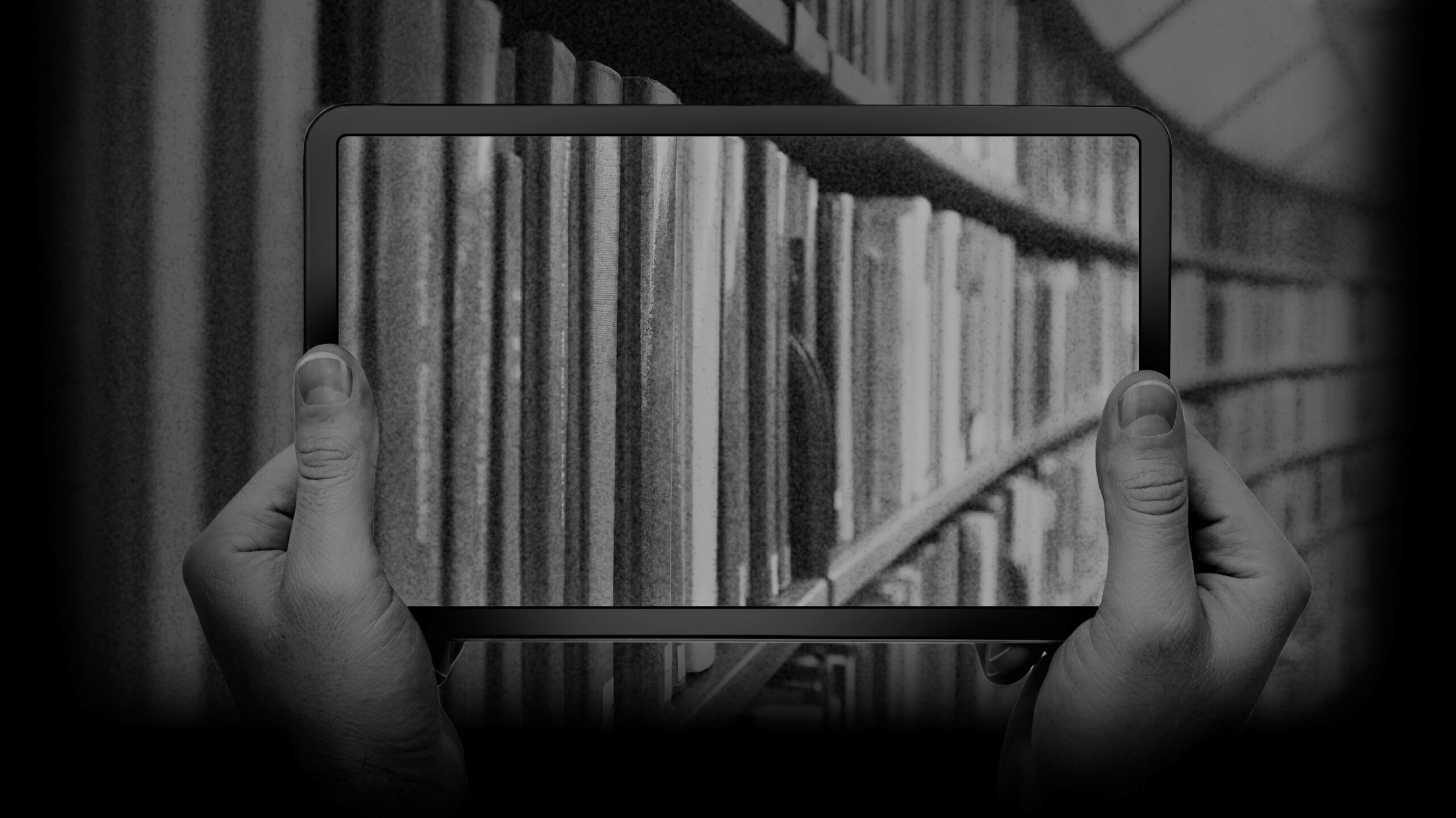
Search
Full Listing
2239 Library Entries
Clear
Search
Type | Title | Year | Author | Links |
---|---|---|---|---|
|
Fighting Hate Speech And Terrorist Propaganda On Social Media In Germany
View Abstract |
2019 | Ritzmann, A. | View Publisher |
|
Fighting ISIS on Facebook – Breaking the ISIS Brand Counter-Narratives Project
View Abstract |
2018 | Speckhard, A., Shajkovci, A. and Bodo, L. | View Publisher |
|
Fighting Terror Online: The Convergence of Security, Technology, and the Law
View Abstract |
2008 | Golumbic, M.C. | View Publisher |
|
Fighting Words: The Persuasive Effect Of Online Extremist Narratives On The Radicalization Process
View Abstract |
2012 | Braddock, K.H. | View Publisher |
|
Fighting, on the Battlefield and Online
View Abstract |
2016 | Carter, A. and Woodruff, J. | View Publisher |
|
Filtering, Blocking and Take-Down of Illegal Content on the Internet
View Abstract |
2015 | Swiss Institute of Comparative Law | View Publisher |