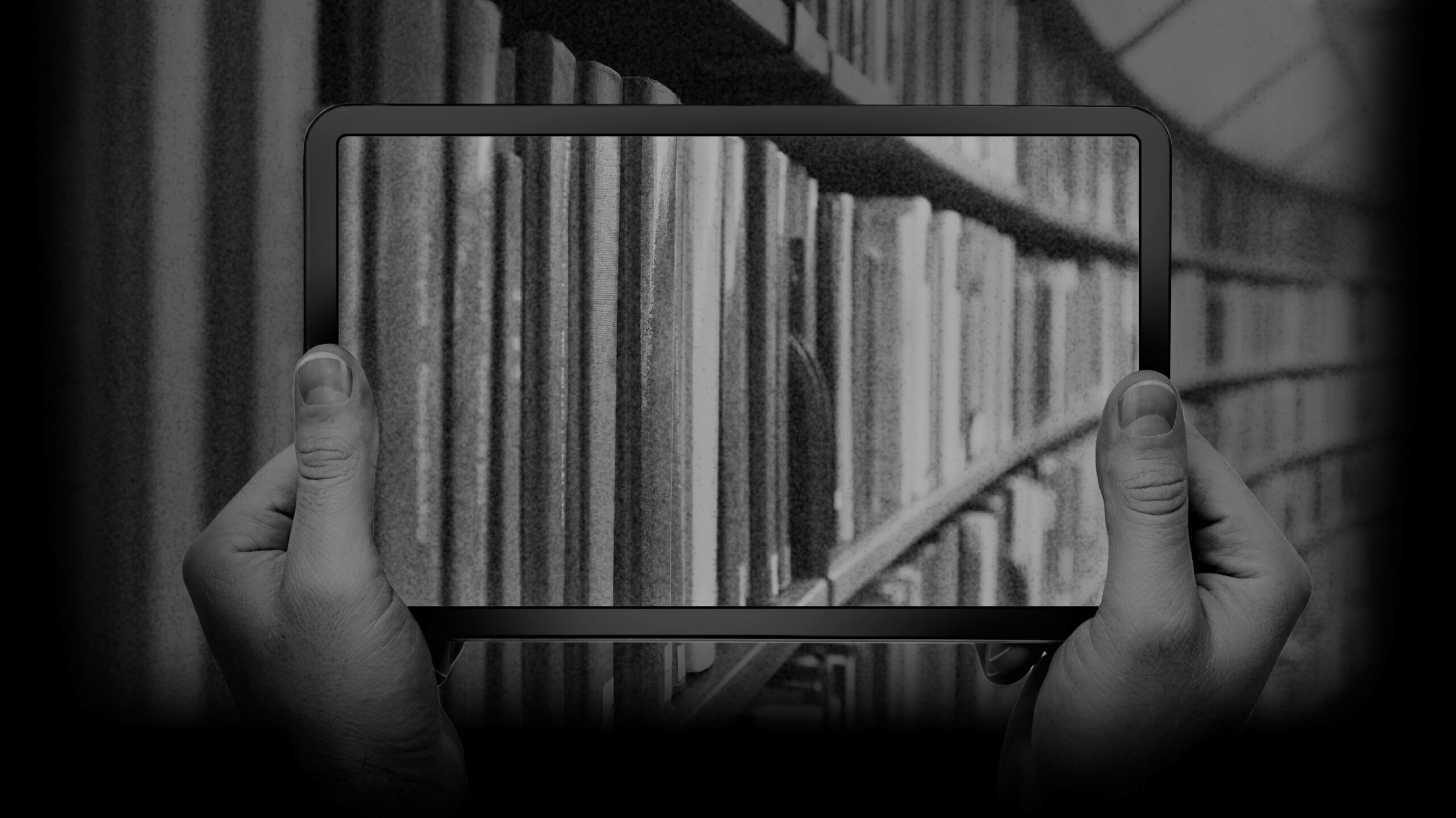
Search
Full Listing
1651 Library Entries
Clear
Search
Type | Title | Year | Author | Links |
---|---|---|---|---|
|
Islamophobia and Twitter: A Typology of Online Hate Against Muslims on Social Media
View Abstract |
2014 | Awan, I. | View Publisher |
|
VOX-Pol Summer School Public Lecture: Brown Moses
View Abstract |
2014 | Higgins, E. | View Publisher |
|
VOX-Pol Inaugural Conference Keynote Lecture: Manuela Caiani
View Abstract |
2014 | Caiani, M. | View Publisher |
|
VOX-Pol Inaugural Conference Keynote Lecture: Thomas Hegghammer
View Abstract |
2014 | Hegghammer, T. | View Publisher |
|
Web as Weapon Internet as a Tool for Violent Radicalization and Homegrown Terrorism ( Part 2 of 2 )
View Abstract |
2014 | Hoffman, B. | View Publisher |
|
Web as Weapon Internet as a Tool for Violent Radicalization and Homegrown Terrorism (Part 1 of 2)
View Abstract |
2014 | Hoffman, B. | View Publisher |