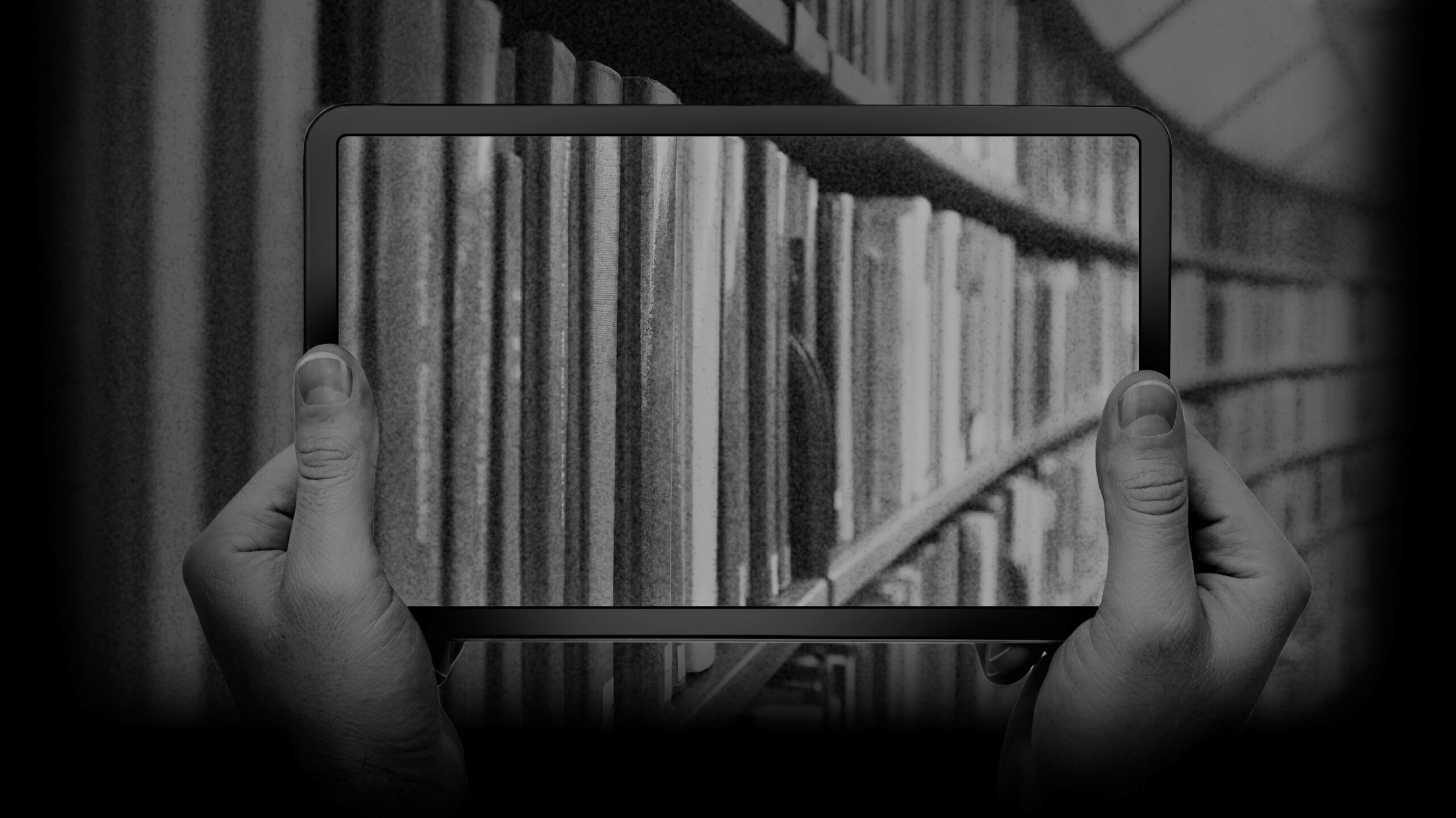
Search
Full Listing
2239 Library Entries
Clear
Search
Type | Title | Year | Author | Links |
---|---|---|---|---|
|
Tracking Online Radicalization Using Investigative Data Mining
View Abstract |
2013 | Wadhwa, P. and Bhatia, M.P.S. | View Publisher |
|
Tracking Online Hate Speech and Identifying Online ‘Raids’ in the UK
View Abstract |
2017 | Online Civil Courage Initiative | View Publisher |
|
Tracking far-right extremist searches in Bosnia & Herzegovina
View Abstract |
2020 | Moonshot CVE | View Publisher |
|
Tracing Transnational Linkages on Twitter: Mapping Indian Diaspora Supporters of Brexit and Trump
View Abstract |
2019 | Leidig, E. | View Publisher |
|
Toxic play: Examining the issue of hate within gaming
View Abstract |
2023 | Munn, L. | View Publisher |
|
Toxic Narratives: Monitoring Alternative-right Actors
View Abstract |
2017 | Baldauf, J., Dittrich, M., Hermann, M., Kollberg, B., Lüdecke, R. and Rathje, J. | View Publisher |