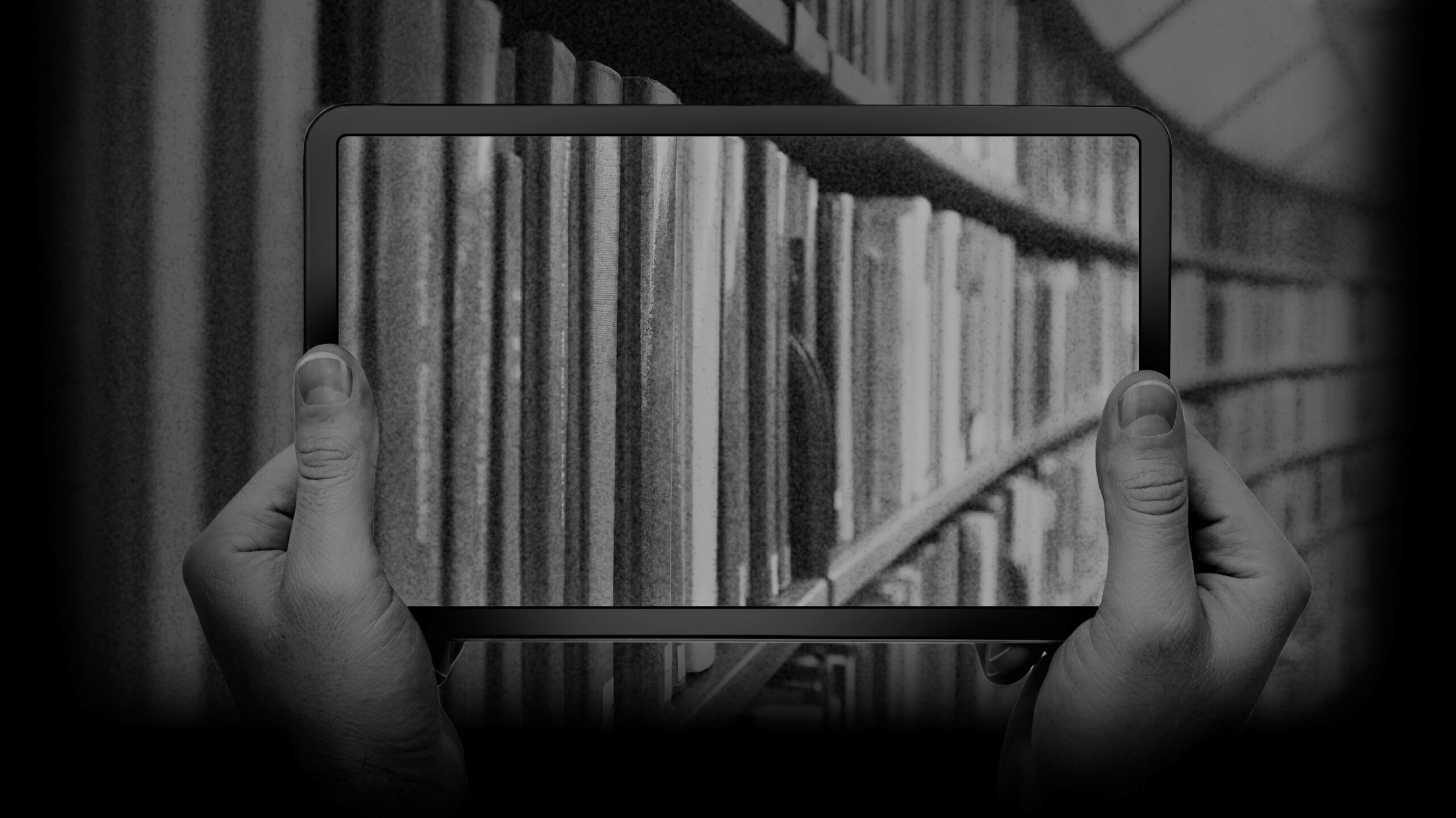
Search
Full Listing
1651 Library Entries
Clear
Search
Type | Title | Year | Author | Links |
---|---|---|---|---|
|
The Supremacy of Online White Supremacists – an Analysis of Online Discussions by White Supremacists
View Abstract |
2015 | Wong, M.A., Frank, R. and Allsup, R. | View Publisher |
|
Golden Dawn, Austerity and Young People: The Rise of Fascist Extremism among Young People in Contemporary Greek Society
View Abstract |
2015 | Koronaiou, A., Lagos, E., Sakellariou, A., Kymionis, S. and Chiotaki-Poulou, I. | View Publisher |
|
The Caliphate Is Not a Tweet Away: The Social Media Experience of Al Qaeda in the Islamic Maghreb
View Abstract |
2015 | Torres-Soriano, M.R. | View Publisher |
|
Inter-ideological mingling: White extremist ideology entering the mainstream on Twitter
View Abstract |
2015 | Graham, R. | View Publisher |
|
Assisting practitioners to understand countering violent extremism
View Abstract |
2015 | Harris-Hogan, S. and Barrelle, K. | View Publisher |
|
Women, Social Media and Violent Extremism
View Abstract |
2015 | Chaudry, R. | View Publisher |