This is the second in a series of four original Blog posts; the first is HERE. [Ed.]
By Matti Pohjonen
The first blog post in this series explored how researchers interested in computational methods can assess the trade-off between the validity of the methods used and the potentially adverse social costs of using them in real-world research situations. It suggested that one way to do this is through what has been called the confusion matrix. This is a research heuristic developed to assess the costs and benefits of research by cross-tabulating the relationship between false positives (e.g. research identifying something as belonging to a category when it should not belong to it) and false negatives (e.g. not being able to identify something into a category when it should belong to it).
It further argued that–in a world where perfectly accurate systems do not exist and where there are always negative externalities to research–this trade-off between the benefits of the methods used and the cost of getting things wrong has to ultimately go beyond methodological considerations alone. This raises the question of ethics.
Building on this approach, this second post in the series is focused on methods and research approaches that have been developed to identify and analyse online violent extremist networks. Underlying this diverse set of methodological approaches is the assumption that any type of activity can be modelled in the form of a network based on the differential relationships found in this activity. What this means in online extremism research is that any type of activity or relationship found online, including on social media–such as likes, shares, comments, retweets, follower and friends–can be represented as a network composed of nodes (e.g. users, posts, comments, tweets, external URLs, or anything that exists as a separate entity in the network) and edges (i.e. the relationships these nodes have with each other). For instance, the network analysis tool ORA used in terrorism research can extract 18 different types of networks from public Twitter data alone.
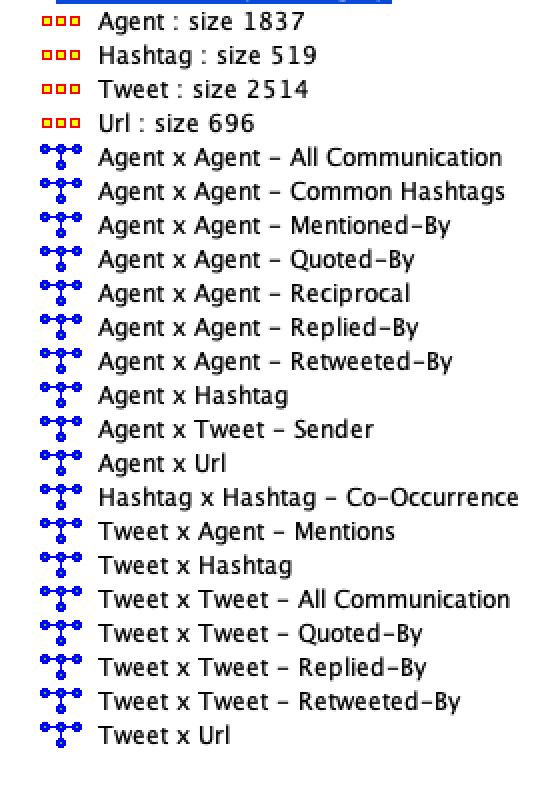
Once the phenomenon in question is represented in the form of a network, researchers can then apply sophisticated statistical tools to gain insights into these networks, including their topological structure, the communities that underlie them, and the different types of actors who compose the network. There are hundreds of metrics developed for this purpose, ranging from centrality metrics (e.g. identifying influential actors in the network) and community detection algorithms (e.g. which actors cluster together based on their activity or how closely knit the communities are) to temporal network models exploring changes in networks over time (e.g. how online communities evolve).
These approaches have been widely used to answer research questions such as the degree of homogeneity of network actors, how related the different communities in them are, key network actors, or even what effect the removal of selected actors will potentially have on the evolution of a network. Furthermore, there are many powerful open-source and commercial software packages available, which facilitate this type of analysis, allowing powerful exploratory visualisation of online and social media data that provide a quick visual overview of the structure of online networks and conversations.
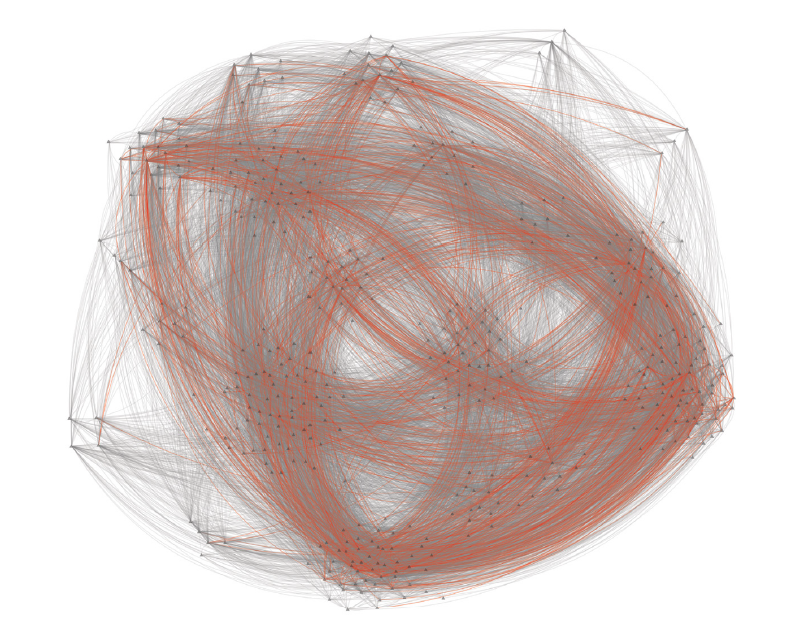
Overview of methods used in online extremism research
Given their flexibility and power, network analysis has been widely used in sociology, political science, and anthropology to explore different types of social phenomena such as organizational structures, kinship, political organisation or community relations. Similarly, in research interested in online extremism, there is a long history of use of network analysis to gain exploratory insight into the actors and communities underpinning extremist groups online, the network dynamics informing extremist activity and changes in them, or modelling the spread and influence of extremist messaging over time.
Some of the earlier influential work on this topic included research into the social networks of the 9/11 attacks, Al-Qaida-linked global Salafist networks, the relationships between members of the Jemaah Islamiyah cell that bombed Bali, and the social networks of the London Bombers and the Madrid train bombers.
More recently, given the growing access to especially public social media conversations and profiles, social network analysis has emerged as a vibrant sub-field of online extremism research used to extrapolate different types of insights from the growing available data. Research using network analysis methods has explored, among other things, the large-scale dynamics of online extremist communities, questions of polarised political discussions during landmark political events, hyperlink relationships between extremist websites, follower or retweet relationships on Twitter, and the relationships between news sources and extremist communities online.
While the social science-oriented research approaches have predominantly used social network analysis as their preferred mode of analysis, other computational approaches have also emerged that combine predictive machine learning with network analysis or explore network behaviour and evolution through computer simulation. Dynamic network analysis or agent-based modelling, for instance, have both developed research approaches that move from more static network relationships to also predict changes in networks over time. Treating social phenomena as a complex system has thus allowed research to explore more experimental research questions such as the hypothetical effect on the overall network structure of removing key actors. Some of the more experimental computer simulations used for this purpose have included techniques such as near-term analysis, or the contagion or epidemic models used to explore, for instance, how misinformation spreads in social media networks over time.
Cost-benefit analysis
While perhaps the most prolific use of network analysis in extremism research was around 10 years ago, these methods remain popular among researchers, especially for their ability to quickly identify and analyse actors and communities online. At the same time, despite their popularity in especially exploratory data analysis and visualisation, criticisms have also been raised about the overall validity of the methods and the potential social costs of using them in especially sensitive social or political situations.
The methodological criticisms raised have argued that these methods often lack a coherent theoretical framework to explain what the often glitzy network visualisations and sophisticated statistical metrics mean. Network analysis excels in more exploratory or descriptive research but it does not always provide a deeper theoretically-grounded understanding of the contextual factors that inform the network dynamics or the actors and communities implicated in them. Focusing only on the network and community metrics can thus risk neglecting complex questions of agency and motivation obviated from the abstract network data. Also, critics have argued that a large portion of the network models used in extremism research have so far been static and thus do not sufficiently take into account constant processes of transformation and change in human networks.
Some of the criticism raised about the social costs have revolved around questions of data privacy and the consequent harm caused to individuals who are falsely identified by the powerful tools available. When lacking the context behind the network data, such approaches can, at worst, lead to false identifications of individuals and communities based on their statistically inferred network relationships. Without careful attention to the context within which these relationships exist, or the social and political factors behind such network dynamics, it is difficult to establish how representative these statistical network dynamics are of violent extremist behaviour more broadly. MacGinty writes that
by its very nature, SNA identifies connections between people. It can help to identify core members of a militant group as well as those on the fringes. It may also identify individuals who are in the social or familial circle of militants but who are completely unconnected with the militant activity. There is a danger that states, through carelessness or an overzealous interpretation of data, target unconnected individuals. Of course, such erroneous targeting can take place with or without assistance from social mapping techniques. Yet, the technocratic imperative of social mapping means that it may fetishise connections between individuals without stopping to ask about the nature of these connections and their political or military significance. The nature of the technology means that analysts may be tempted to identify connection after connection, potentially criminalising an entire community. How data is used is as important as its collection, and states may be tempted to pursue a ‘guilt by association’ route, especially in contexts in which human rights are regularly abused.
Network analysis-based approaches thus provide researchers with one of the most powerful research tools to explore online activity, but this activity needs to be contextualised with more grounded empirical domain knowledge assessing how relevant the visualisations and statistics are for the research questions. When assessing the costs and benefits of using network analysis for research on violent online extremism, researchers need to thus carefully consider how to best balance the ability of these approaches to extrapolate quick and powerful insights with more contextual factors that help interpret the results and validate them. One possible way forward to mitigate this challenge of context and interpretability is to include more mixed-method elements in the research, such as interviews, digital ethnography, or content analysis, to ground these analyses.
Matti Pohjonen is a Researcher for a Finnish Academy-funded project on Digital Media Platforms and Social Accountability (MAPS) as well as a VOX-Pol Fellow. He works at the intersection of digital anthropology, philosophy and data science. On Twitter @objetpetitm.
Previously in the Series:
Part I: A Framework for Researchers
Next in the series:
Part III: Analysing Extremist Content
Part IV: Predicting Extremist Behaviour